Getting started with Oracle Cloud Generative AI using Python
Did you know Oracle Cloud Infrastructure hosts LLMs for Meta Lllama and Cohere Command-R. If you have an OCI subscription (not Free Tier) you can interact with them in the Generative API Playground. The models are only available in select regions, so you need to be subscribed to one of Brazil East (Sao Paulo), Germany Central (Frankfurt). Japan Central (Osaka), UK South (London) or US Midwest (Chicago).
See the Pretrained Foundational Models in Generative AI documentation for the latest list of supported models and regions.
For this post I'm going to take a quick look at interacting with these models directly with a simple Python client using the OCI Python SDK.
Python SDK
First we'll setup a separate Python environment for this playground and install the OCI Python SDK and OCI CLI
$ python3 -m venv venv
$ source venv/bin/activate
$ pip install oci oci-cli
The following assumes you have your API access credentials in your local ~/.oci/config
file. A quick way to get started is run oci setup bootstrap
. If you are using an alternative authentication method like session tokens or instance principles you will need to adjust the code below accordingly.
Create a client and get the list of available models
First we'll create a GenerativeAiClient to list the available models.
import oci
config = oci.config.from_file(profile_name="DEFAULT")
config["region"] = "uk-london-1"
generative_ai_client = oci.generative_ai.GenerativeAiClient(config=config)
list_models_response = generative_ai_client.list_models(
config["tenancy"],
lifecycle_state="ACTIVE",
)
Filter the results to get just the available CHAT
models.
for model in list_models_response.data.items:
if model.capabilities == ['CHAT']:
print(model.display_name)
meta.llama-3.3-70b-instruct
meta.llama-3.2-90b-vision-instruct
cohere.command-r-08-2024
meta.llama-3.2-11b-vision-instruct
cohere.command-r-plus-08-2024
meta.llama-3.1-70b-instruct
meta.llama-3.1-405b-instruct
cohere.command-r-plus
cohere.command-r-16k
meta.llama-3-70b-instruct
Building our first chat request
Now we can use one of the available models to build a chat interaction. The OCI Generative AI APIs have different clients for the Meta Llama and Cohere models. Lets start with using the meta.llama-3.3-70b-instruct
model.
We'll use the GenericChatRequest
to construct the LLM request. The API follows a common style of setting a collection of one or more user and assistant messages. A full context can be built up with the history of interactions. For this example we just set the initial user message, which is passed into a chat request with optional parameters to modify the model temperature, seed, etc. The full set of available options are covered in the API documentation
prompt = "Why is the sky blue. Explain in one sentenace."
messages = [oci.generative_ai_inference.models.UserMessage(
role="USER",
content=[oci.generative_ai_inference.models.TextContent(
type="TEXT",
text=prompt,
)]
)]
serving_mode = oci.generative_ai_inference.models.OnDemandServingMode(
serving_type="ON_DEMAND",
model_id="meta.llama-3.3-70b-instruct"
)
chat_request = oci.generative_ai_inference.models.GenericChatRequest(
api_format="GENERIC",
messages=messages,
is_stream=False,
temperature=0.7,
)
chat_details = oci.generative_ai_inference.models.ChatDetails(
compartment_id=config["tenancy"],
serving_mode=serving_mode,
chat_request=chat_request,
)
inference_client = oci.generative_ai_inference.GenerativeAiInferenceClient(config=config)
response = inference_client.chat(chat_details=chat_details)
For this simple example response streaming is disabled, so the response returns a single payload once the generation is complete. We'll covering streaming responses below, but first lets take a look at the returned response.data
.
{
"chat_response": {
"api_format": "GENERIC",
"choices": [
{
"finish_reason": "stop",
"index": 0,
"logprobs": {
"text_offset": null,
"token_logprobs": null,
"tokens": null,
"top_logprobs": null
},
"message": {
"content": [
{
"text": "The sky appears blue because when sunlight enters Earth's atmosphere, it is scattered in all directions by tiny molecules of gases such as nitrogen and oxygen, with shorter, blue wavelengths being scattered more than longer, red wavelengths.",
"type": "TEXT"
}
],
"name": null,
"role": "ASSISTANT",
"tool_calls": []
}
}
],
"time_created": "2025-03-28T11:26:38.971000+00:00"
},
"model_id": "meta.llama-3.3-70b-instruct",
"model_version": "1.0.0"
}
Some of the key items are the chat_response.choices[0].finish_reason
which indicates if the response completed normally, or encountered an issue like hitting the max tokens length. The actual text of the response is returned in chat_response.choices[0].message.content[0].text
Streaming responses
A more common interaction style we're used to seeing with LLMs is getting a real-time streaming response rather than waiting for the full generation to complete.
To enable streaming we simply update the is_stream
option in the chat request.
chat_request = oci.generative_ai_inference.models.GenericChatRequest(
...
is_stream=True,
...
)
Now we need to change to way we handle the response data to output each response chunk from the event stream
import json
response = inference_client.chat(chat_details=chat_details)
for event in response.data.events():
chunk = json.loads(event.data)
if "message" in chunk:
print(chunk["message"]["content"][0]["text"], end="")
else:
print(chunk)
Using the Cohere models
To use the Cohere Command-R models is very similar but uses the CohereChatRequest
for the request data structure
prompt = "Why is the sky blue. Explain in one sentenace."
serving_mode = oci.generative_ai_inference.models.OnDemandServingMode(
serving_type="ON_DEMAND",
model_id="cohere.command-r-08-2024"
)
chat_request = oci.generative_ai_inference.models.CohereChatRequest(
message=prompt,
response_format=oci.generative_ai_inference.models.CohereResponseTextFormat(),
is_stream=True,
)
chat_details = oci.generative_ai_inference.models.ChatDetails(
compartment_id=config["tenancy"],
serving_mode=serving_mode,
chat_request=chat_request,
)
inference_client = oci.generative_ai_inference.GenerativeAiInferenceClient(config=config)
response = inference_client.chat(chat_details=chat_details)
for event in response.data.events():
chunk = json.loads(event.data)
if "text" in chunk:
print(chunk["text"], end="")
References
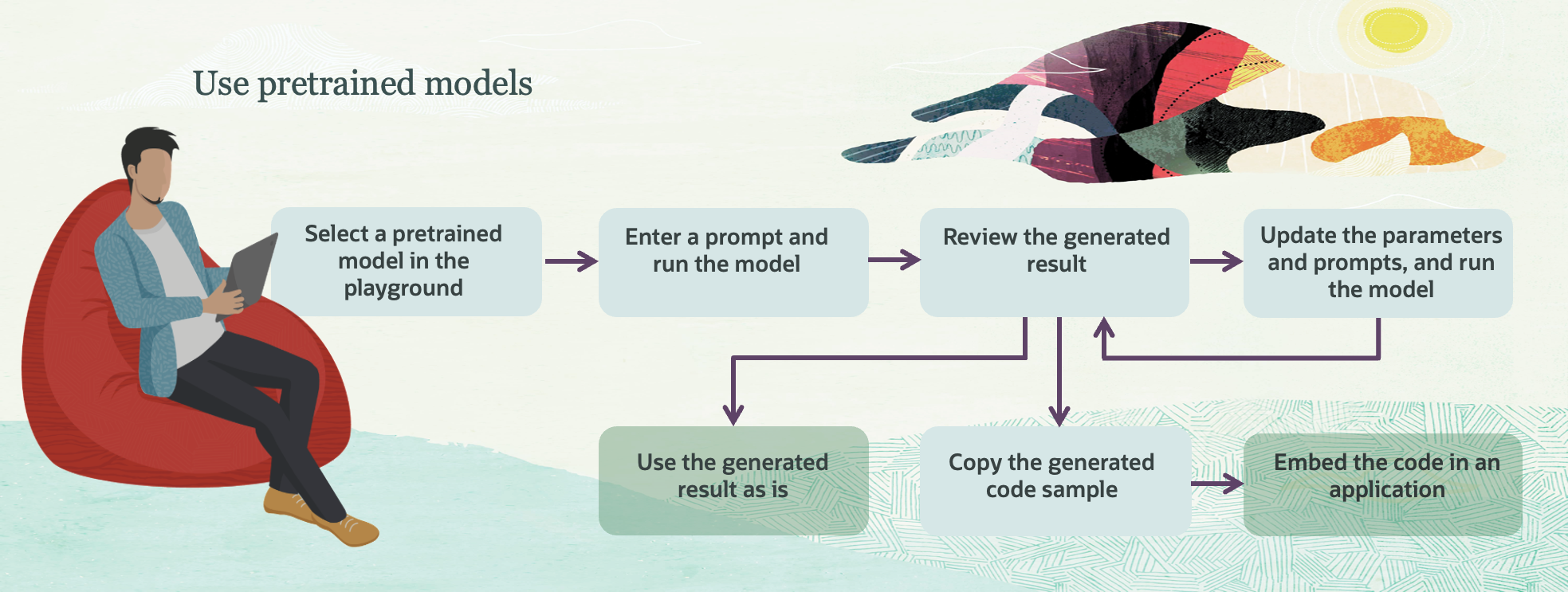